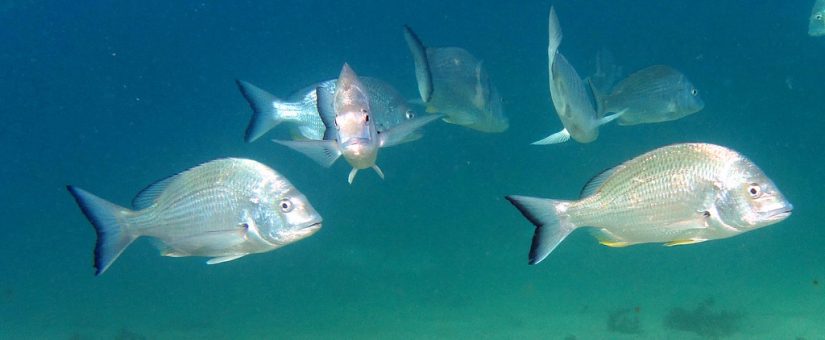
Computer Vision Resources: Fish Classification Datasets
- Posted by Ellen Ditria
- On June 17, 2020
by Sebastian Lopez
Computer vision is transforming the collection and processing of digital imagery for ecology and conservation. In aquatic environments, computer vision tools for automatic fish identification are heavily sought after, but robust and open-access fish datasets are hard to find. Here, I share some of the most used, open-access and updated fish datasets for automatic fish detection and classification.
Computer vision
Computer vision is the scientific field that develops and trains computers to understand and interpret objects from digital images or videos. Computer vision can automatically detect, count and even track objects from digital photos.
In ecology and conservation, computer vision is transforming information processing by quickly and accurately analysing the vast amount of digital imagery collected by researchers through previous decades.
Computer vision in aquatic ecosystems
In aquatic environments, one of the most sought-after computer vision tools is a platform that can automatically identify and detect fish species from underwater footage. Since cameras are becoming more common to monitor and study fish populations, researchers are developing tools that can reduce the tedious and time-consuming task of manually analysing the footage.
An automatic tool that streamlines the process and produces results more quickly than humans would be an important achievement for the monitoring of aquatic ecosystems.
Computer vision tools require data
Developing automatic tools for fish detection require LARGE amounts of data. Many aquatic researchers own enormous fish datasets; however, most of these datasets have
- restricted availability
- fish have not been manually annotated and labelled
What makes an excellent fish computer vision dataset?
A great dataset for a fish computer vision tool requires
- sufficient images of all the fish to be identified
- the user already knows the IDs of all the fish
- the fish have been outlined (mask or bounding box) along with its label
- the dataset has a variety of environmental conditions (i.e. high and low visibility footage)
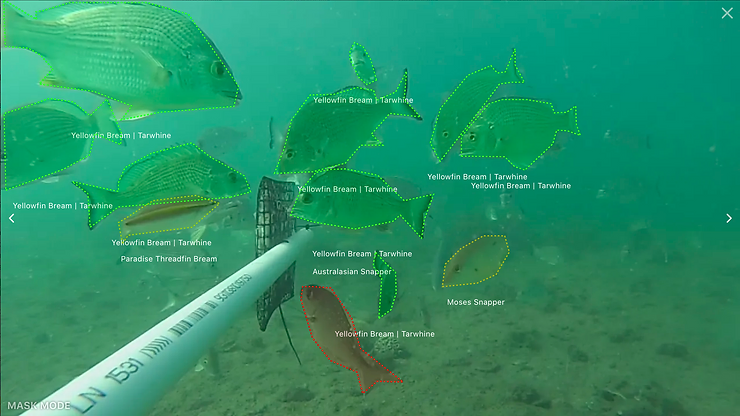
While it is difficult to find datasets with these needs, here are some publically available datasets of fish imagery for computer vision tasks. These datasets have been used in several peer-reviewed computer vision/fish classification studies and can help the development of a fish computer vision tool.
Datasets
- Extensive datasets from 4 NOAA programs. Include still or video imagery of benthic fish and invertebrates across different locations, depths and backgrounds.
- The dataset includes 3,960 images collected from 468 species across different backgrounds and illuminations.
- Extensive collection of ~80k fish crops and ~45k bounding box annotations for a wide variety of fish species.
Fish4Knowledge (Fish Detection)
- A large and well-known ground-truth dataset with 1700 minutes of fish footage.
Fish4Knowledge (Fish Species Recognition)
- Dataset used in LifeClef 2015 competition.
- 20 manually annotated videos, 15 fish species to support the learning of fish recognition models.
DIDSON data for fish classification
- Dual-Frequency Identification Sonar (DIDSON), fishery acoustic observation data of 8 fish species from the USA.
- Fish-Pak: an image dataset of 6 different fish species, captured by a single camera from different pools located nearby the Head Qadirabad, Chenab River in Punjab, Pakistan.
Leave a comment if you know of any other computer vision fish datasets.
Follow the progress of #FishID; an automatic platform for fish species identification and abundance quantification through this blog or @seabassphd.
0 Comments